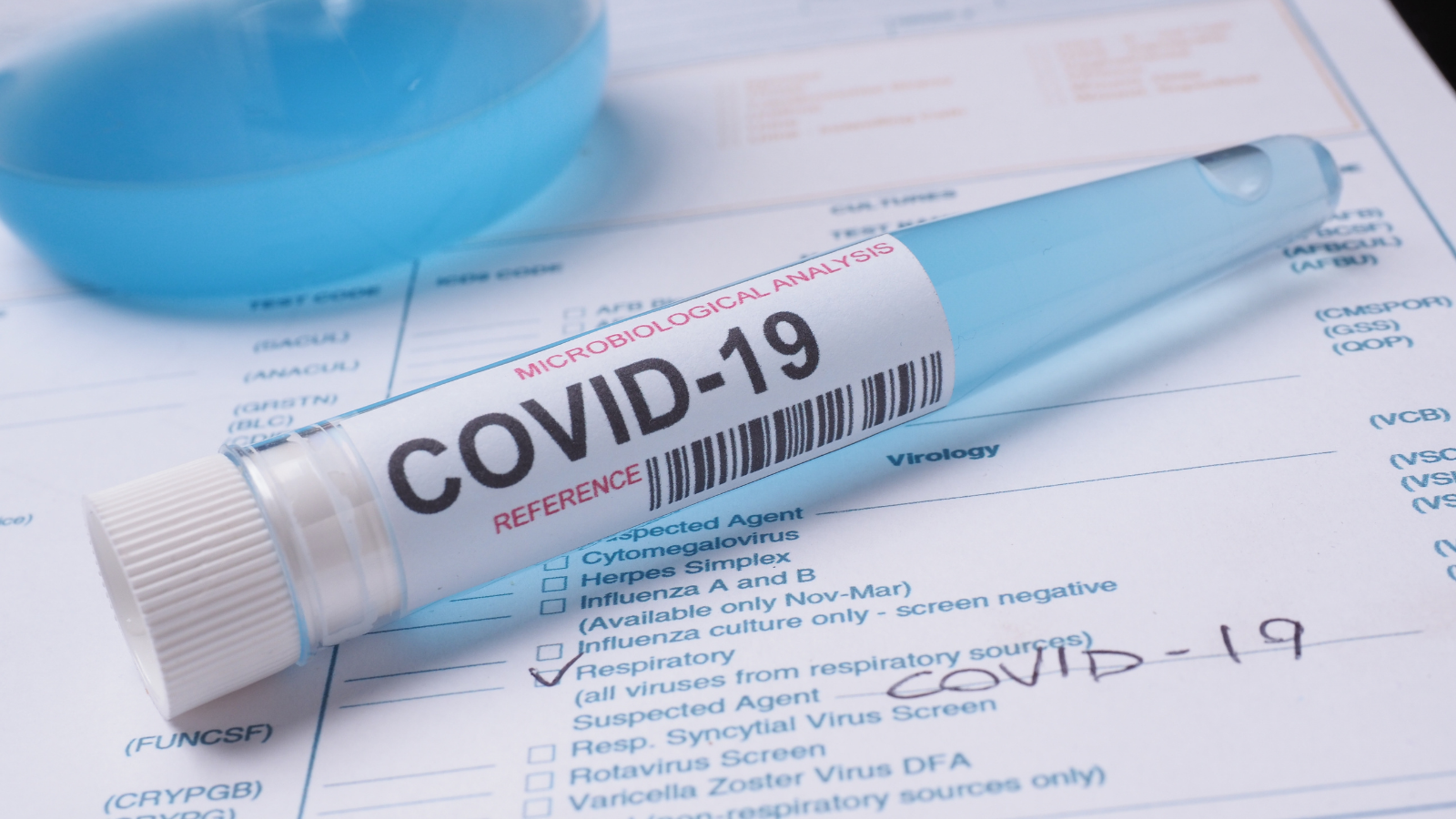
COVID-19 is a heterogenous disease that continues to evolve. As the world faces new waves of the pandemic, unique populations are not responding to the virus in the same way. These differences in biological host responses of infected individuals have been difficult for scientists to fully understand. To help inform effective patient management and public health policy making, Dr. Fei Wang, associate professor of population health sciences; Dr. Rainu Kaushal, senior associate dean for clinical research at Weill Cornell Medicine and chair and professor of population health sciences; Dr. Yongkang Zhang, research associate in population health sciences; Dr. Mark Weiner, professor of clinical population health sciences; Dr. Chang Su, postdoctoral associate in population health sciences at the time of the study; Dr. James Flory, assistant professor of population health sciences; and Dr. Edward J. Schenck, assistant professor of clinical medicine, derived clinical subphenotypes for COVID-19 patients. This npj Digital Medicine study was different from previous subphenotype studies of COVID-19 as it focused on a larger, more representative, and diverse patient population.
The researchers used machine learning and clinical data to analyze New York City patients with confirmed cases of COVID-19 treated in emergency departments or inpatient settings between March 1 and June 12, 2020. The 14,418 patients were from five major medical centers within the INSIGHT Clinical Research Network database. Of this cohort, four reliable subphenotypes were characterized. Subphenotype I is mild and consists of 33.02 percent of the patients. These patients are younger, have less abnormal blood test results and less comorbidity burden, and they are associated with better clinical outcomes in terms of 60-day mortality rate, mechanical ventilation rate, and ICU admission rate. Subphenotypes II (37.16 percent of patients) and III (18.12 percent of patients) are moderate, but with distinct characteristics. Where Subphenotype II is associated with higher inflammation but lower comorbidity burden, Subphenotype III is associated with higher comorbidity burden but lower inflammation. Subphenotype III has higher 60-day mortality rate and ICU admission rate, but lower mechanical ventilation rate, compared to Subphenotype II. Subphenotype IV is severe and consists of 11.7 percent of the patients. These patients are older, with more abnormal blood test results, higher comorbidity burden, and the worst clinical outcomes in 60-day mortality. This group also has more disadvantaged social determinants of health, such as vulnerable socioeconomic neighborhood status.
Because these subphenotypes were highly predictive of clinical outcomes and the researchers were able to determine the association of social determinants of health, these findings could benefit clinical trial development and reduce social disparities in the pandemic moving forward. The researchers suggest that data collection outside of New York City could help generalize these subphenotypes further.
- Highlights