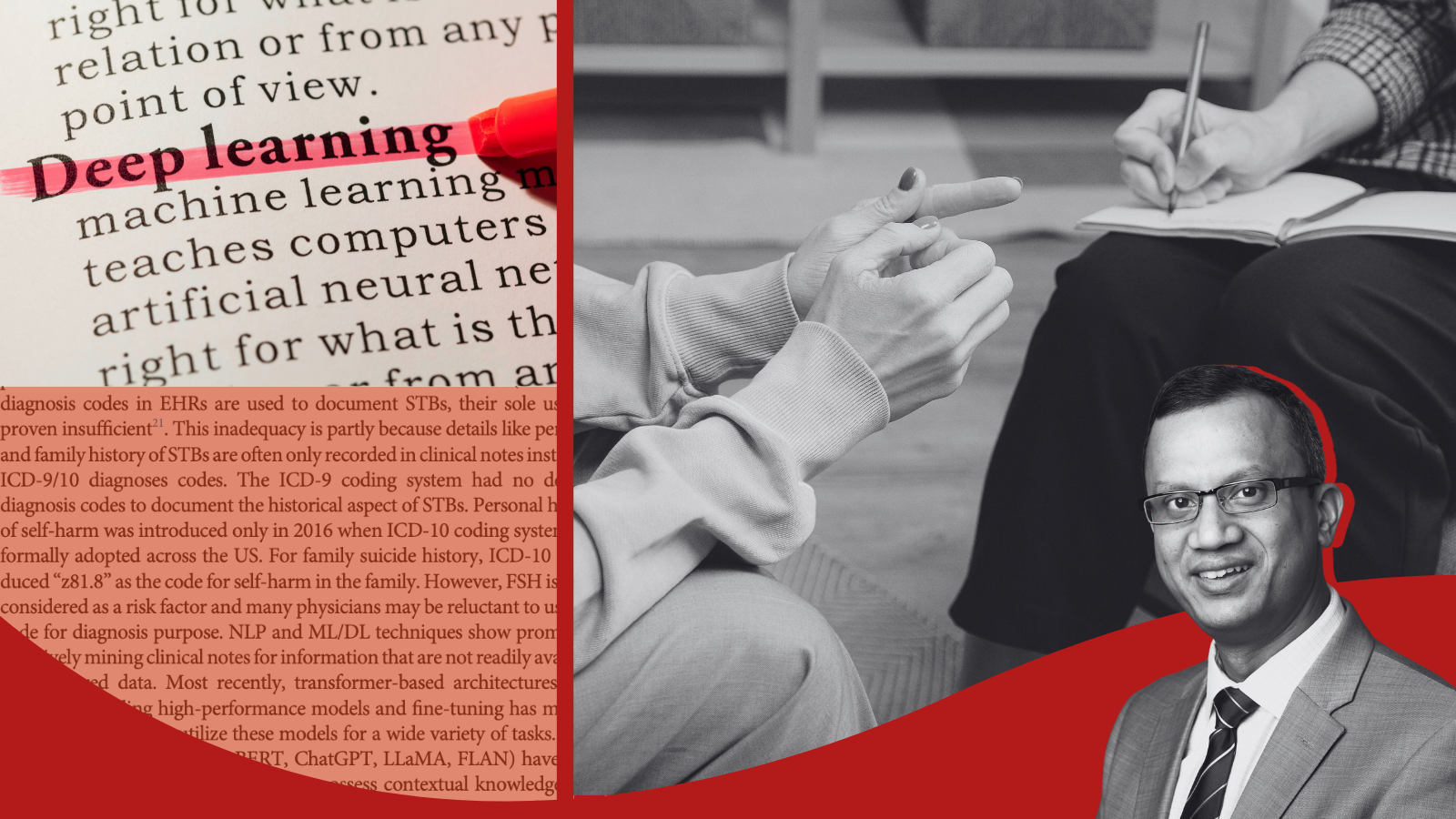
Suicide is a critical public health challenge, ranked as the tenth leading cause of death overall in the US. Personal (PSH) and family history (FSH) of suicidal thoughts and behaviors are significant risk factors associated with suicide. While these behaviors provide critical insight into predicting and preventing suicidal thoughts and behaviors (STBs), there is limited research on the automatic identification of such information from patient electronic health records.
In a study in npj Digital Medicine, Dr. Jyotishman Pathak, chief of the Division of Health Informatics and the Frances and John L. Loeb Professor of Medical Informatics, and colleagues developed natural language processing (NLP), and deep learning (DL) algorithms aimed at detecting PSH and FSH from clinical encounter notes. The algorithms were developed and validated at three academic medical centers: Weill Cornell Medicine, Northwestern Medicine, and the University of Florida Health.
Results indicate that traditional methods of structured information collection using diagnosis codes might miss up to 90 percent of patients exhibiting STBs, which are often only documented as unstructured data during a clinical encounter. The algorithms developed in the study effectively extracted this information for about 80 percent of cases. In particular, the DL methods demonstrated higher performance than expert-driven, rule-based NLP methods in detecting both PSH and FSH. Altogether, the study highlights the significant potential of both NLP and DL tools in identifying personal and family histories of STBs.
- Highlights