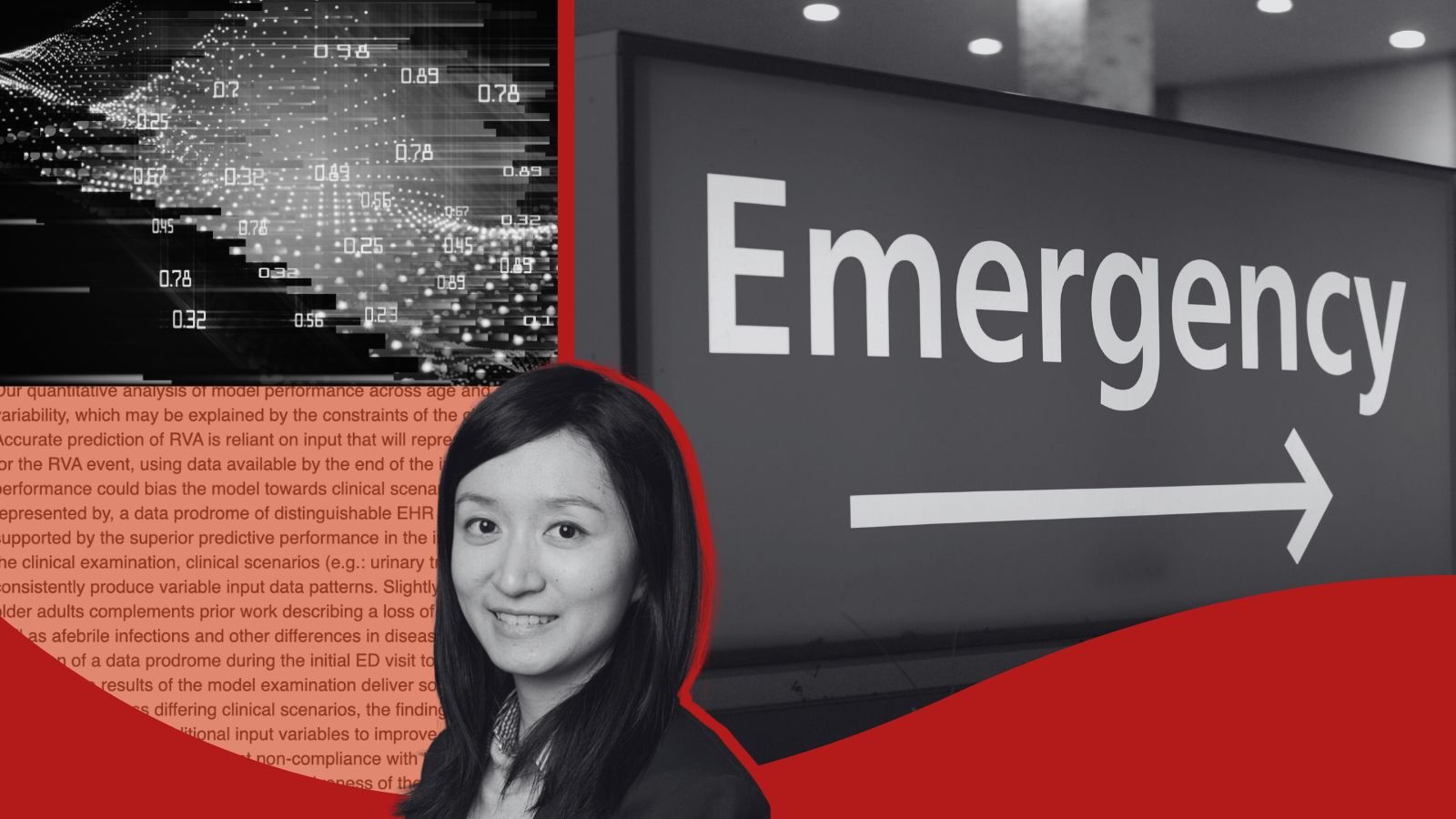
Emergency department (ED) return visit admissions (RVA) occur when patients discharged from the ED return to the ED within a short period, requiring hospital admission. Over half a million US ED visits annually are followed by RVA within 72 hours of the initial ED visit. The process is associated with adverse outcomes, including increases in mortality, higher need for surgical intervention, and longer hospitalizations.
Because the information on ED is heterogeneous, predicting RVA is a technical challenge. To address this issue, a study in PLOS Digital Health, led by Dr. Yiye Zhang, associate professor of population health sciences and population health sciences in emergency medicine, and colleagues, uses machine learning to develop a predictive model for RVA.
The model drew data from electronic health records at three urban EDs. Findings indicate that the machine learning approach is predictive for ED RVA and fairly generalizable but requires clinical actionability. The complexity of the model creates challenges to integration in clinical settings and could be improved by providing outputs that directly support clinical decision-making. The study highlights the potential value of predictive analytics in emergency medicine and can inform future model development that is explainable to clinicians.
“This publication is from our National Institute on Aging-funded R01 with Dr. Peter Steel, associate professor of clinical emergency medicine, and colleagues in the Emergency Medicine Department at WCM,” said Dr. Zhang. “This work will serve as a preliminary study which we will build upon, including methodology extension and implementation studies.”
- Highlights