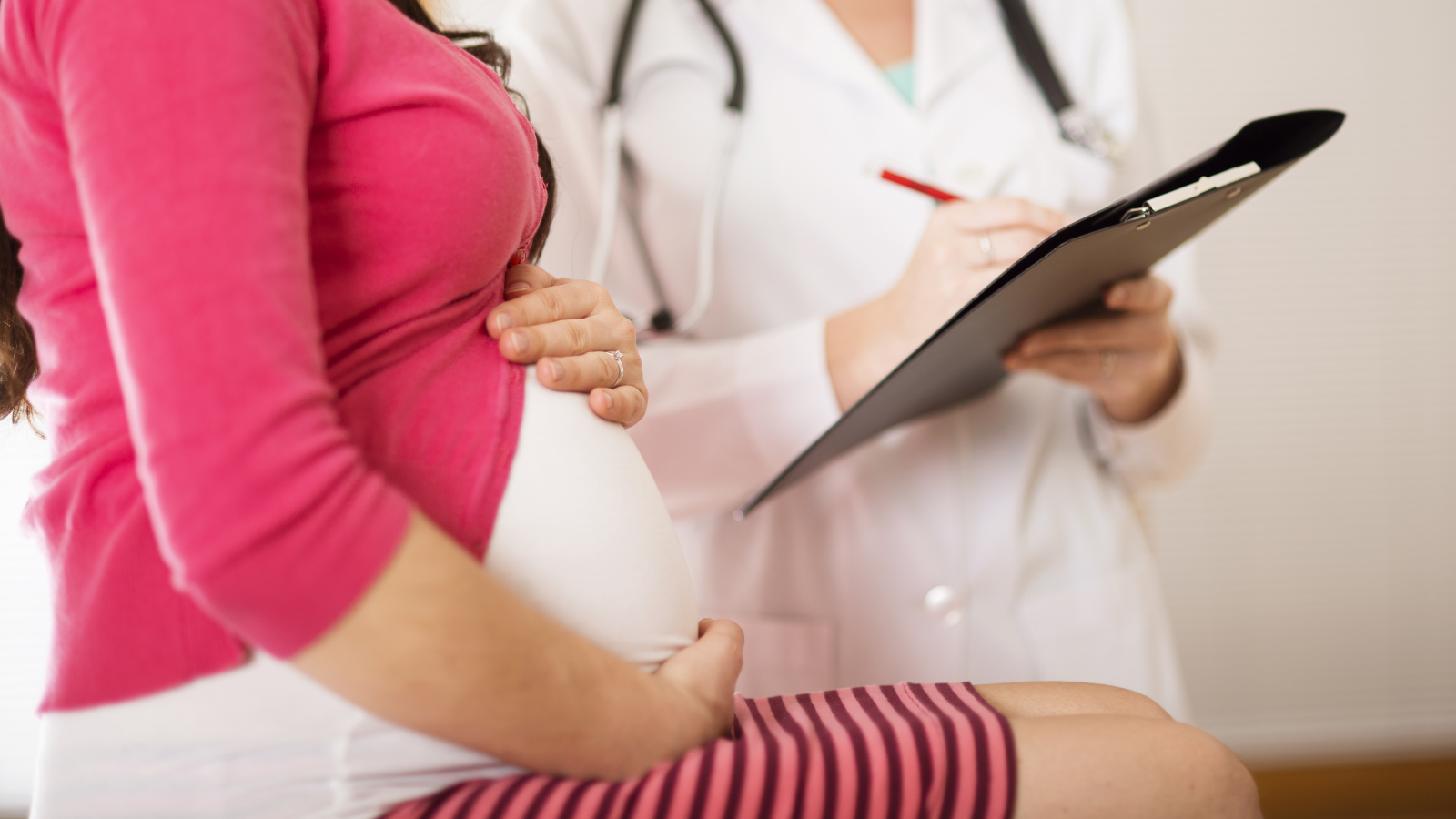
According to the Centers for Disease Control and Prevention, about 1 in 8 women experience symptoms of postpartum depression (PPD) nationally. However, there is a lack of tools that can predict PPD. In a new Journal of Affective Disorders study, Weill Cornell Medicine’s Yiye Zhang, PhD, MS, assistant professor of population health sciences, and Jyotishman Pathak, PhD, professor of population health sciences, joined colleagues to create a machine learning framework for PPD risk prediction. Two electronic health records (EHRs) datasets containing data on 15,197 women from 2015 to 2018 at a single site, and 53,972 women from 2004 to 2017 at multiple sites were used as development and validation sets to construct the model. A minimal list of features from the EHR datasets was used to ensure model performance and to enable future point-of-care risk prediction, with a primary outcome of a diagnosis of PPD within one year following childbirth. The researchers concluded that EHRs and machine learning can help identify women at risk for PPD early in their pregnancies, which may facilitate scalable and timely prevention and intervention that could reduce negative outcomes and the associated burden.
- Highlights