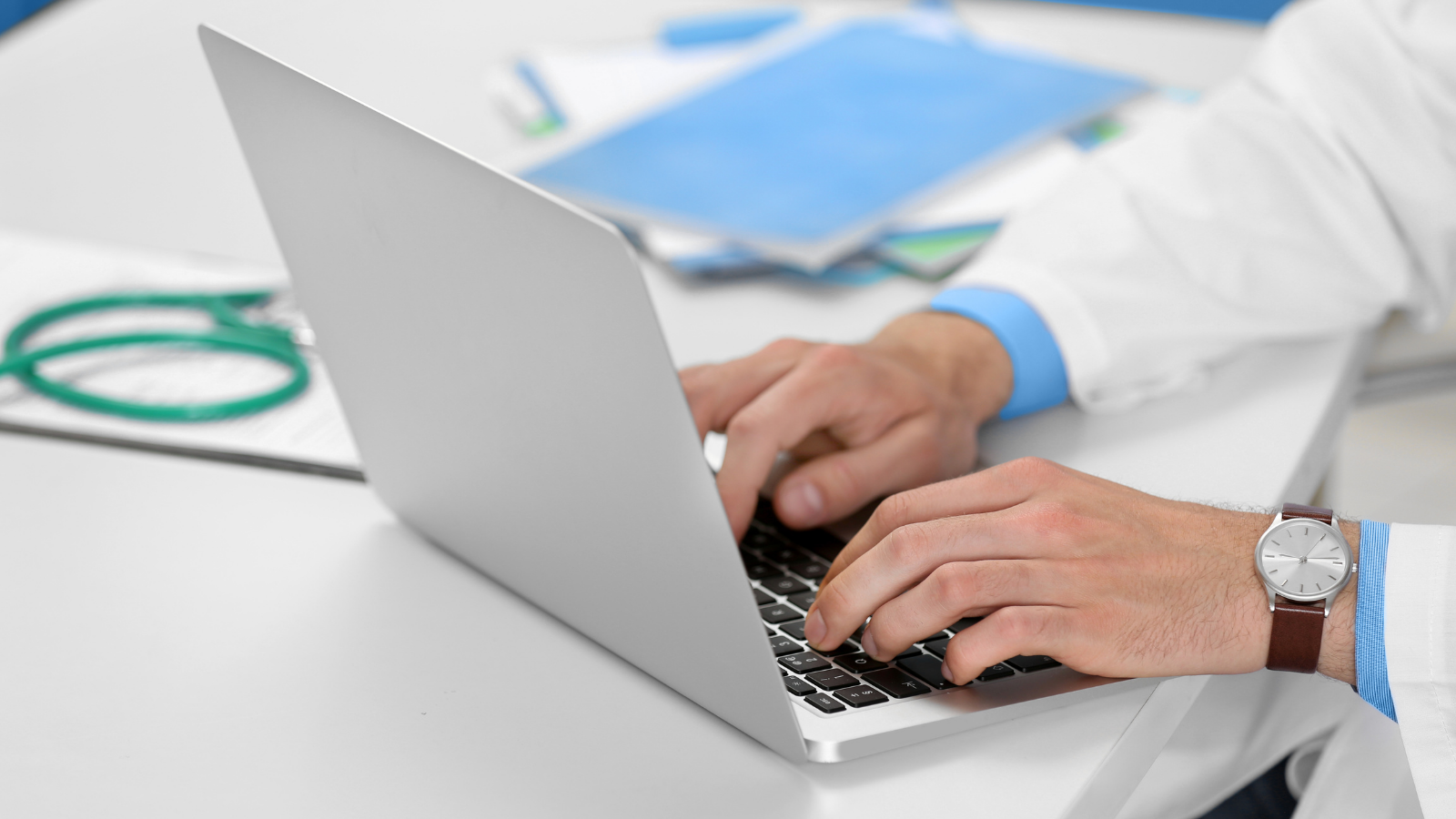
While social determinants of health (SDoH) impact patient risks and clinical outcomes, this nonclinical information is typically locked in unstructured clinical notes. However, leveraging SDoH has the potential to improve diagnosis, treatment planning, and patient outcomes across populations. In a new Journal of the American Medical Informatics Association study, senior author Jyotishman Pathak, PhD, professor of population health sciences; first author Braja Patra, PhD, MTech, research associate in population health sciences; Thomas Campion, PhD, MS, associate professor of population health sciences; Mark Weiner, MD, professor of clinical population health sciences; Mohit Sharma, MPH, research manager in population health sciences; Veer Vekaria, research assistant in population health sciences; and colleagues completed a systematic review of the state-of-the-art natural language processing (NLP) approaches and tools that focus on identifying and extracting SDoH data from unstructured clinical text in electronic health records (EHRs). Using three scholarly databases, the researchers reviewed 82 publications. They found that smoking status, substance use, homelessness, and alcohol use are the most frequently studied SDoH categories. Machine learning approaches are popular for identifying smoking status, substance use, and alcohol use, while homelessness and less-studied SDoH like education, social isolation, and family problems were more commonly identified using rule-based approaches. Overall, the researchers concluded that NLP has significant potential in extracting SDoH data from clinical notes, which can help develop screening tools, risk prediction models, and clinical decision support systems.
- Highlights