Dr. Fei Wang, associate professor of population health sciences at Weill Cornell Medicine, consistently works with innovative models and concepts to advance his research. Recently, Dr. Wang has collaborated on multiple projects to understand the best uses for federated learning, a general learning strategy that can be applied to any type of concrete machine learning model.
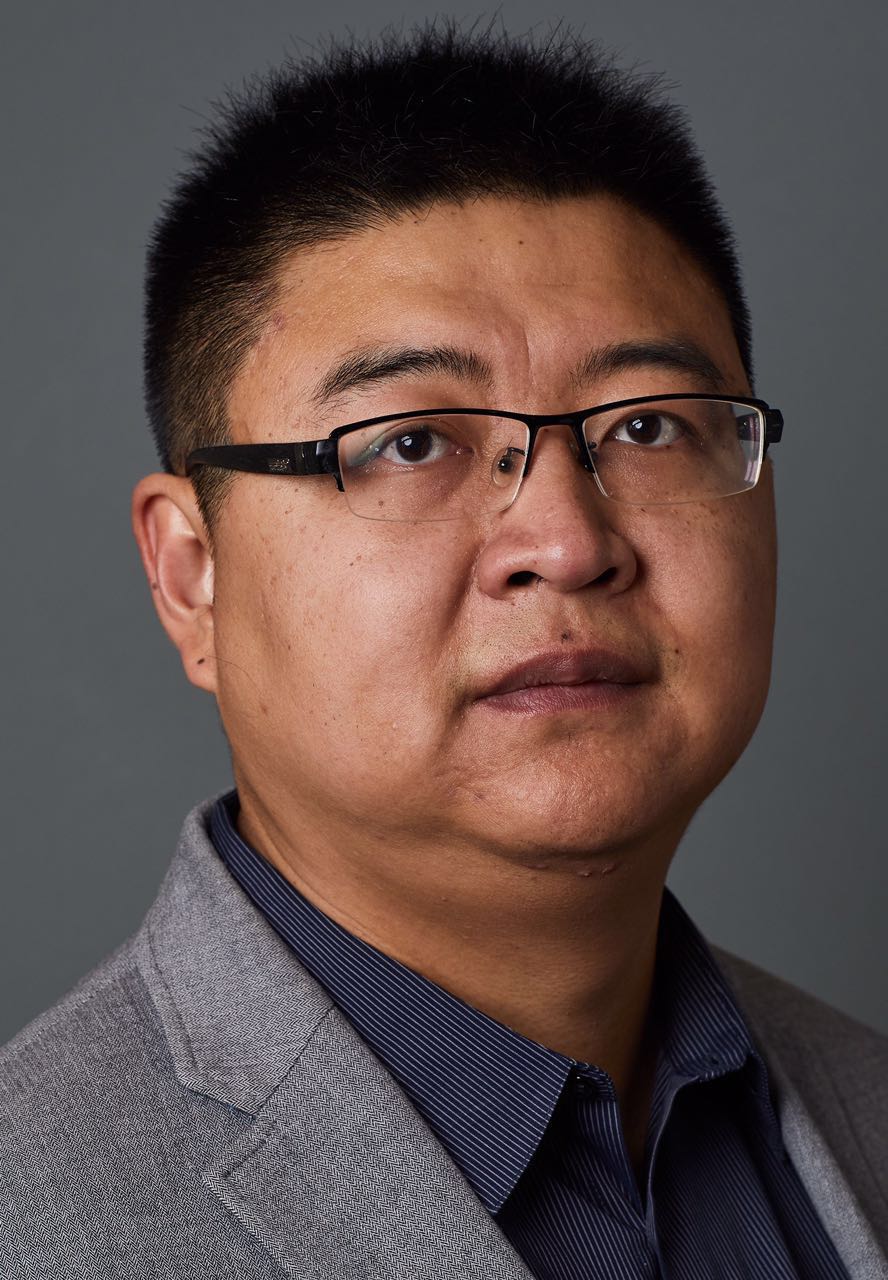
Dr. Fei Wang
In a recent study Dr. Wang collaborated on with Mount Sinai colleagues, the researchers explored a federated learning strategy which leverages patients’ information across the five hospitals in the Mount Sinai Health System. Their goal was to build a machine learning model to predict the seven-day mortality rate for hospitalized COVID-19 patients based on their demographics and clinical information.
According to Dr. Wang, federated learning creates a secure way of leveraging patient information located at different places to train a unified model. Therefore, it has the potential to incorporate more diverse data than other strategies.
While the researchers found that federated learning worked better than local models in this particular study, there are caveats scientists must keep in mind for other studies.
“Intuitively, federated learning can securely leverage more information across multiple local sites, thus, the model can be trained with more data. But actually, the patient populations across different local sites may have different characteristics,” Dr. Wang said. “In that case, integrating two very different data sets may worsen the performance of the local predictor trained on each local data set. Therefore, it is critical to be mindful of the potential discrepancies among the sample distributions across different local sites when designing the federated learning algorithm.”
This could be useful for collaborative clinical research networks in the future. With each network composed of multiple local clinical research institutions, federated learning creates an opportunity to build a global computational model by leveraging patient information from local institutions without needing to physically integrate raw patient records. “However, it is important for the data at all local sites to be harmonized with common data models, such as OMOP or PCORnet for electronic health records,” Dr. Wang said.
The strategy could be implemented across broader areas of the healthcare system, as well.
“Given the fragmented nature of the healthcare system and sensitivity of patient data, federated learning definitely provides a promising solution as it aims to leverage information from different local sites in a privacy-preserving way,” Dr. Wang said.
In addition, Dr. Wang would also like to consider different strategies for information aggregation. Because local institutions will ultimately be adopting the model, performance at these local sites is the most important.
“I’m thinking the key should be how to leverage the information from other local sites to improve the model performance on each local site. In other words, building better locally customized models, rather than a global consensus model,” Dr. Wang said.
There are other key federated learning research aspects that Dr. Wang would like to pursue moving forward, as well.
One factor is decentralized learning. Dr. Wang and colleagues have begun to explore this strategy in a preprint.
“Federated learning still assumes the local sites need to communicate with a central server to transmit model parameter updates, which may increase the communication burden, as well as make the central server vulnerable to potential attacks,” said Dr. Wang. To avoid that, using a “pure” decentralized learning strategy allows the local sites to communicate with only each other, without a central server.
Dr. Wang would also like to explore additional indices. Currently, federated learning mostly looks at model performance. However, other aspects like how model fairness (the role that biases and model interactions play in outcomes) will change with federated learning compared with local training, is not clear. How to comprehensively consider these different aspects of the model with the federated learning strategy is an important research topic.
A review of federated learning for health informatics has been published by Dr. Wang and colleagues to open discussion of the strategy for future studies.
The Department of Population Health Sciences addresses the intersection of health and practice. Serving as a collaborative and interdisciplinary hub for clinical research, the Department aims to improve the health of populations and reduce inequities through applied research, technological innovations and novel education programs.
- Highlights