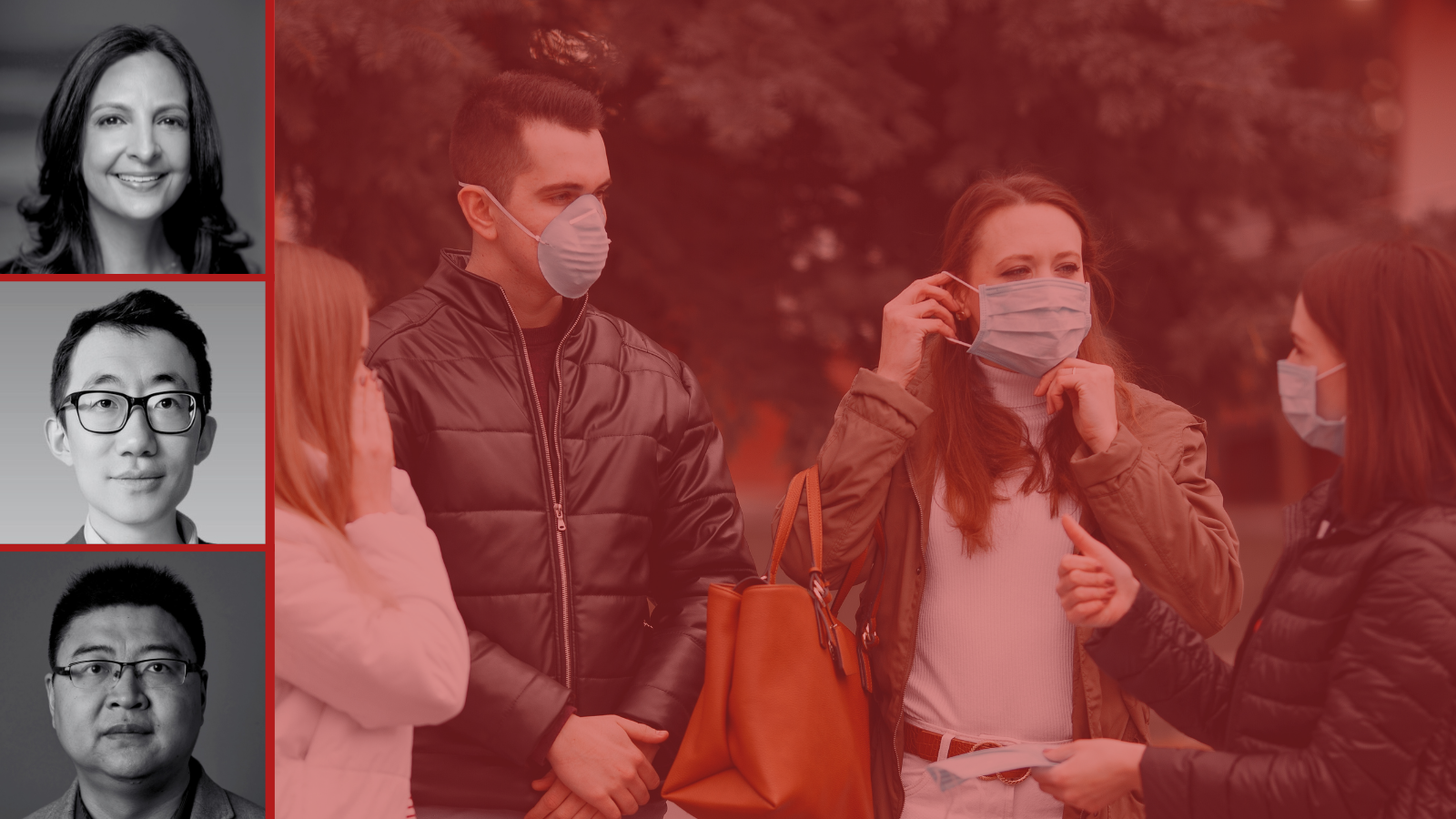
Growing evidence indicates the existence of post-acute and long-term effects of COVID-19, called post-acute sequelae of SARS-CoV-2 infection (PASC), or long COVID. However, few studies have developed machine learning tools to identify risk factors that might make someone more likely to develop long COVID. This may be attributed to the heterogeneity of PASC conditions or small sample sizes of patient data.
To address these challenges, a data-driven study in Communications Medicine led by Dr. Rainu Kaushal, senior associate dean for clinical research and chair of population health sciences, Dr. Fei Wang, professor of population health sciences and founding director of the Institute of AI for Digital Health (AIDH), and Dr. Chengxi Zang, instructor in population health sciences, investigates the predictability of certain long COVID conditions and their associated factors. The study was conducted as part of the NIH Researching COVID to Enhance Recovery (RECOVER) Initiative.
Researchers used two large electronic health record (EHR) cohorts from the PCORnet clinical research networks to analyze both COVID-infected and non-infected adults from March 2020 to November 2021. EHRs include INSIGHT, covering ~1.4 million patients in the NYC area, and OneFlorida+, covering ~0.7 million patients from Florida.
Results indicate that severe acute infections, being underweight, and baseline conditions such as cancer or cirrhosis are likely associated with increased risk of long COVID conditions.
“Long COVID includes a series of conditions involving many organ systems with varying severities,” Dr. Wang said, “We found that different conditions were associated with different predictabilities. For example, severe conditions such as dementia, heart failure, and kidney failure were more predictable, while mild conditions such as fatigue, and headache were less predictable.”
Researchers also developed machine learning-based prediction models to identify patients more likely to experience long COVID conditions based on their baseline characteristics and acute severity of COVID infection. While there are ongoing challenges to defining less predictable PASC diagnoses and managing heterogeneous PASC conditions, this study demonstrates prospective solutions.
“We found that if information was available from when a person first started having symptoms, it was easier to predict if that person would develop health problems connected to long COVID,” said Dr. Zang. “These can include malnutrition and chronic obstructive pulmonary disease. Our findings suggest the potential use of machine learning to help identify some patients who are at risk of long COVID.”
- Highlights