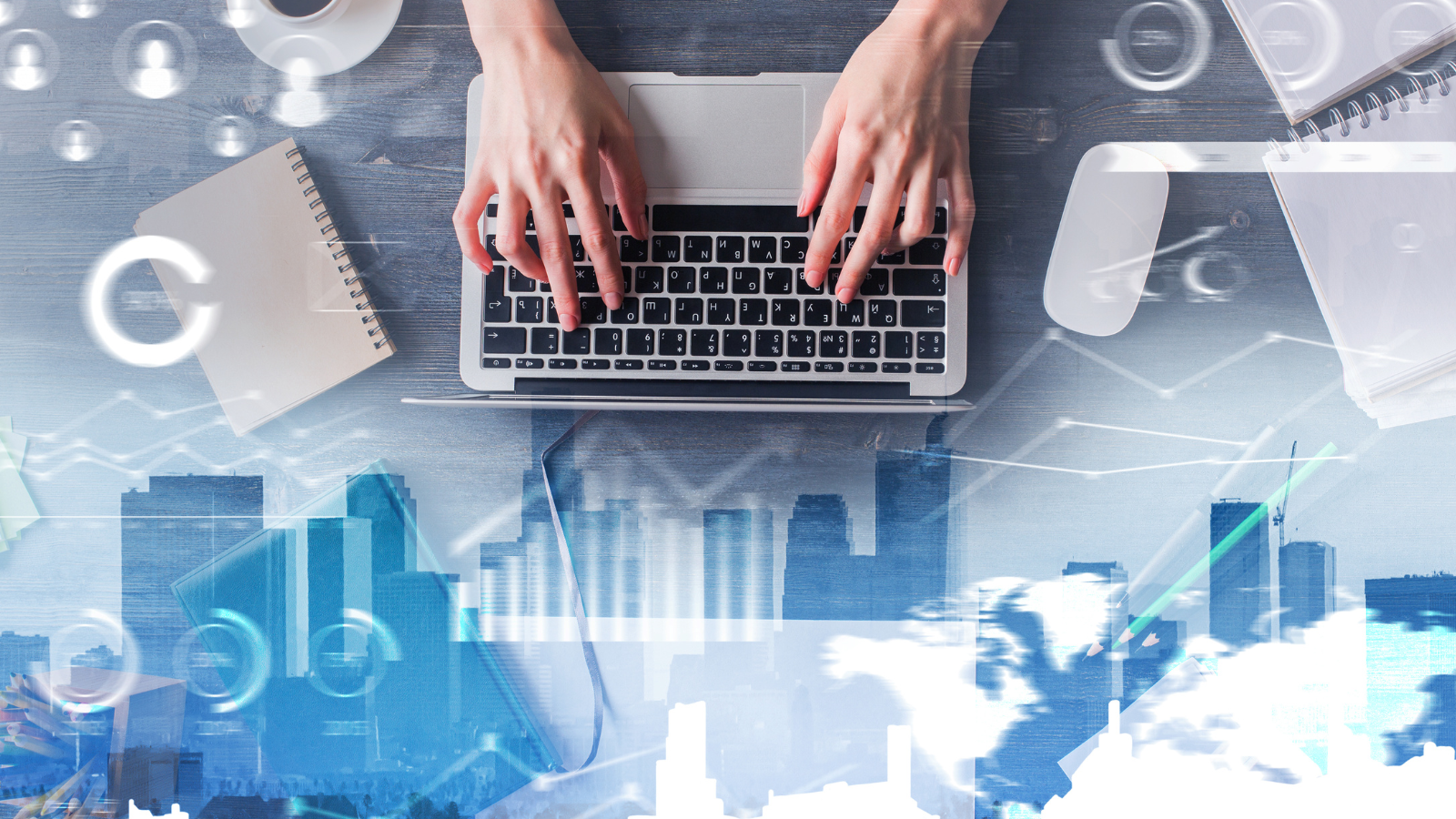
At the 2023 NVIDIA GPU Technology Conference (GTC), NVIDIA CEO Jensen Huang shared information about the artificial intelligence (AI) technology affecting every industry. He unveiled BioNemo: a service from NVIDIA that provides state-of-the-art generative models for drug discovery. Among the nine models is MoFlow, a generative chemistry model developed by Dr. Fei Wang, associate professor of population health sciences, and Dr. Chengxi Zang, instructor in population health sciences.
“We proposed the model in 2020, when we didn’t even have the term ‘generative AI,’” said Dr. Zang. “It was a very niche, novel, and brave idea. BioNemo now features a suite of models for protein structure prediction, biomolecule representation, biomolecule generation, and molecular docking. Ours is one of two for biomolecule generation.”
Per their paper, MoFlow is a flow-based model that generates molecular graphs. These graphs represent information that is relevant to molecular structure, and can be used to visualize chemical reactions, identify the properties of a compound, and as used in MoFlow, aid in AI-driven drug discovery. Dr. Zang explains that a normalizing flow-based model is one type of deep generative model, which can learn and sample from complex data distributions. As such, their model attempts to capture the chemical space—comprised of an estimated 1060 molecules—to generate bonds and atoms that can be assembled into a chemically valid molecular graph.
The model offers exact and likelihood training and efficient one-pass embedding and generation; the implication of these merits is efficiency in model training and inference. The model also provides chemical validity guarantees, meaning that the model can generate realistic molecular graphs. MoFlow was initially validated through a series of tasks and is regularly tested to ensure consistent accuracy and performance over time. In the process of drug discovery and development, which can be both costly and time-consuming, MoFlow allows researchers to generate models in automatic and intelligent ways aimed at drug optimization.
“Broadly speaking, all of our efforts use advanced technology, including AI and machine learning, to achieve better healthcare,” said Dr. Zang. “In our lab, we have developed ways to better define diseases, like we’re doing with long COVID through the RECOVER initiative. We identify groups who are at-risk and create digital prevention strategies by focusing on the whole drug discovery pipeline. MoFlow is especially useful at the start of that pipeline.”
Dr. Zang is grateful to Dr. Wang for his collaboration on the development of MoFlow, and is part of Dr. Wang’s recently launched Institute of Artificial Intelligence for Digital Health, where he continues his work with machine learning and AI. In the future, he wants to bridge any gaps between technology, clinicians, and patients, such that clinicians and patients are more actively involved in and aware of the technology used in their care.
He also appreciates the support of Dr. Rainu Kaushal, senior associate dean for clinical research and chair of the Department of Population Health Sciences, and Dr. Jyotishman Pathak, chief of the Division of Health Informatics and Frances and John L. Loeb Professor of Medical Informatics, who he describes as part of a collaborative, encouraging, and fast-paced group.
“We’re exploring cutting-edge research topics and methods,” he said. “Our teams move very quickly, and we have a good sense of how to use new technology in an otherwise rigorous and traditional business. We will continue to work in ways that support biomedical research and patient care.”
- Highlights