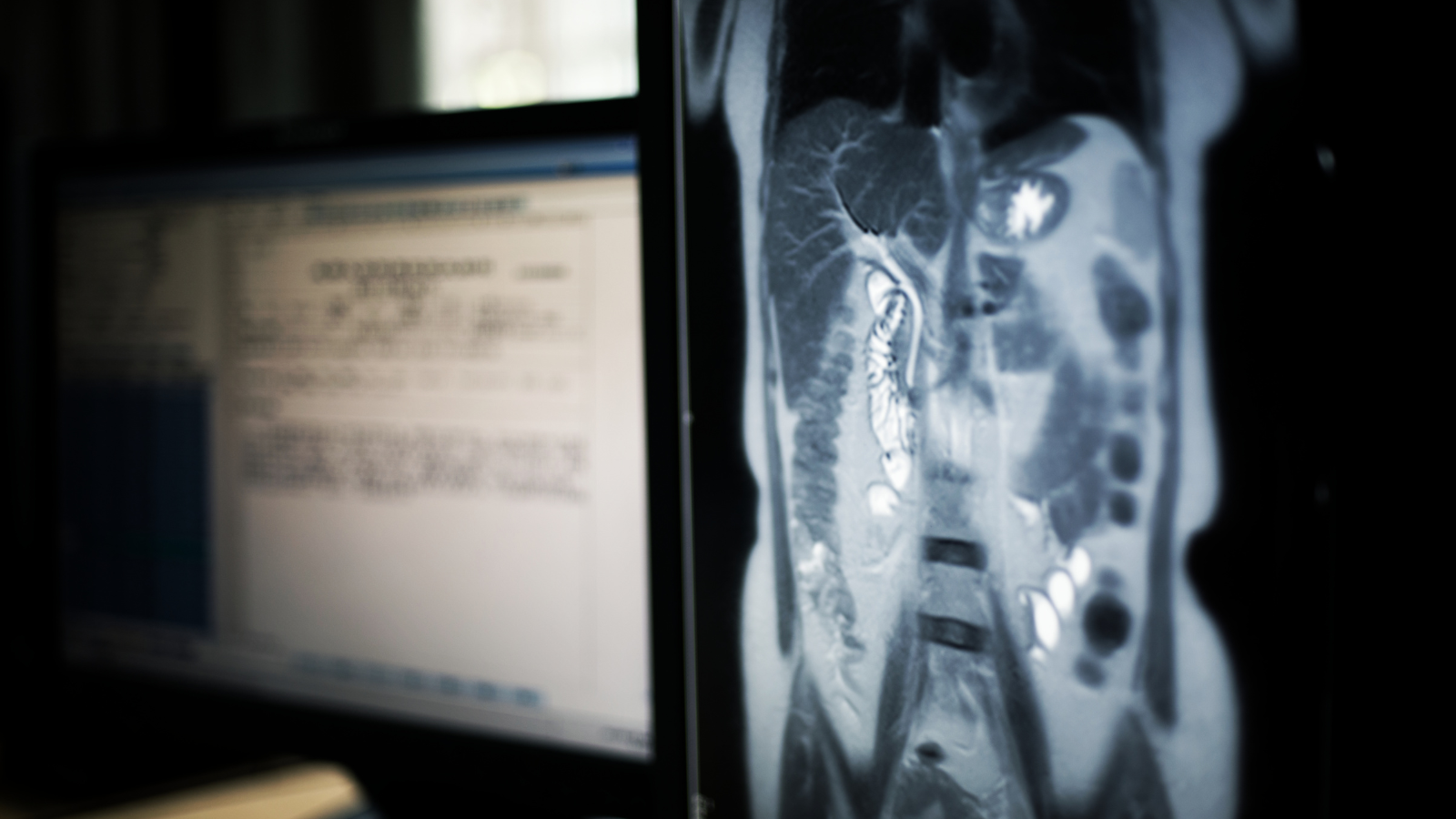
Medical images are common diagnostic and prognostic tools in patient care. In clinical practice, manual examination of these images is labor-intensive and prone to error. Technology including convolutional neural networks (CNNs) have shown performance at or above the human specialist level on a series of medical image diagnosis tasks. However, CNNs are vulnerable to “noise” (irrelevant or corrupted discriminative information) that is undetectable to humans, posing significant security risks and challenges. In a new study published in Pattern Recognition, Dr. Yifan Peng, assistant professor of population health sciences at Weill Cornell Medicine, and colleagues found that noise in medical images might be a key contributor to performance deterioration of CNNs, as noisy features are learned unconsciously by CNNs. They propose a novel defense method by embedding sparsity denoising operators in CNNs for improved robustness. After testing various attacking methods on two medical image modalities, they found that their proposed method can successfully defend against those unnoticeable adversarial attacks. They believe these findings are critical for improving and deploying CNN-based medical applications in real-world scenarios.
- Highlights