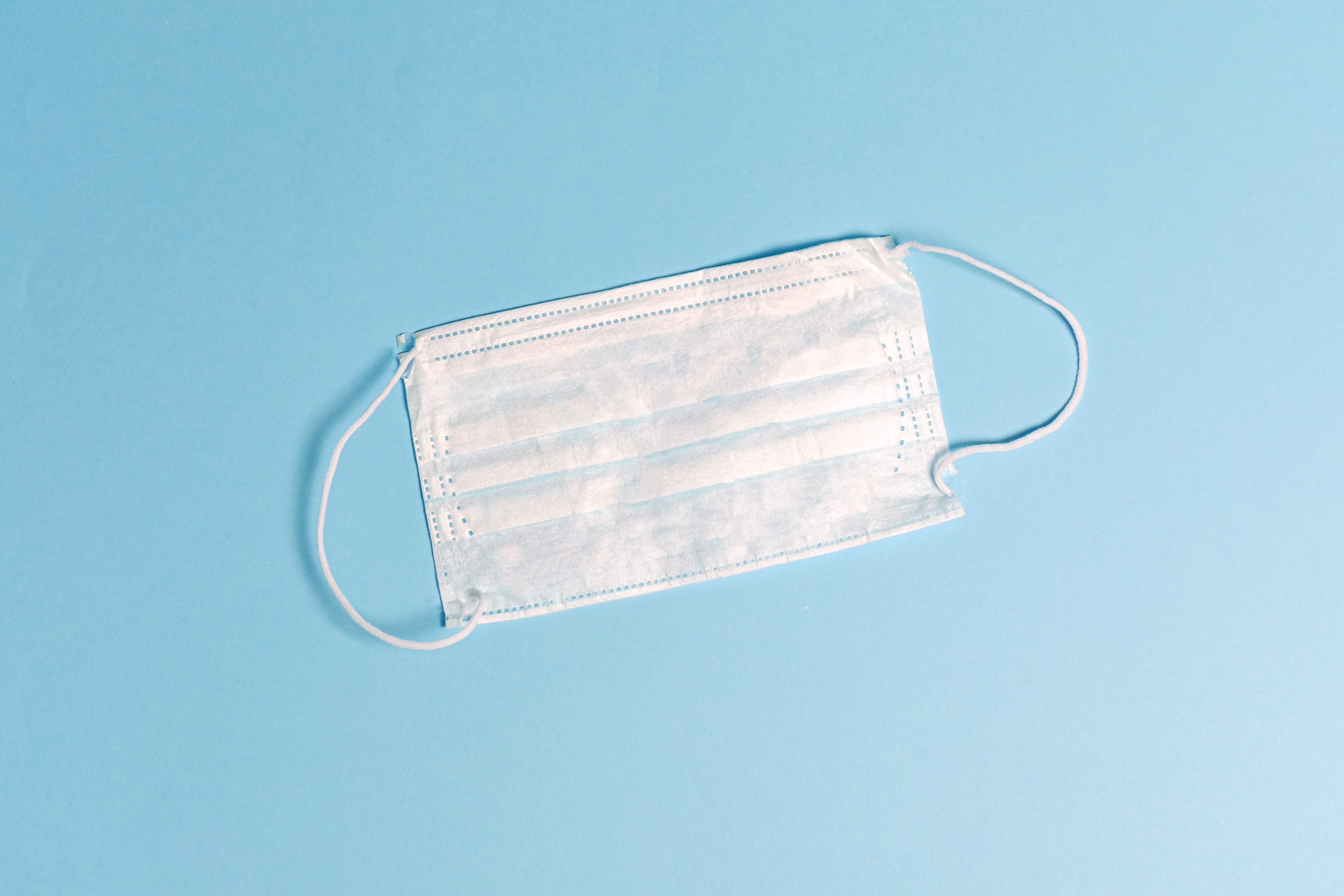
In a new article published in Science Translational Medicine, Dr. Nathaniel Hupert, associate professor of population health sciences and medicine at Weill Cornell Medicine, worked with colleagues to show how influenza-like illness (ILI) outpatient surveillance data can be used to estimate the prevalence of SARS-CoV-2 in the United States. To date, detection of the COVID-19 virus has relied heavily on reverse transcription polymerase chain reaction (PT-PCR) testing, which has limited availability and high false-negative rates. Dr. Nathaniel and his fellow researchers found a surge of non-influenza ILI above the seasonal average in March 2020, which helped them build a conceptual model for the COVID-19 pandemic characterized by a rapid spread across the country. With over 80% of infected patients remaining undetected, the researchers stress the importance of using seroprevalence data and syndromic surveillance for early detection and understanding of emerging infectious diseases.
- Highlights