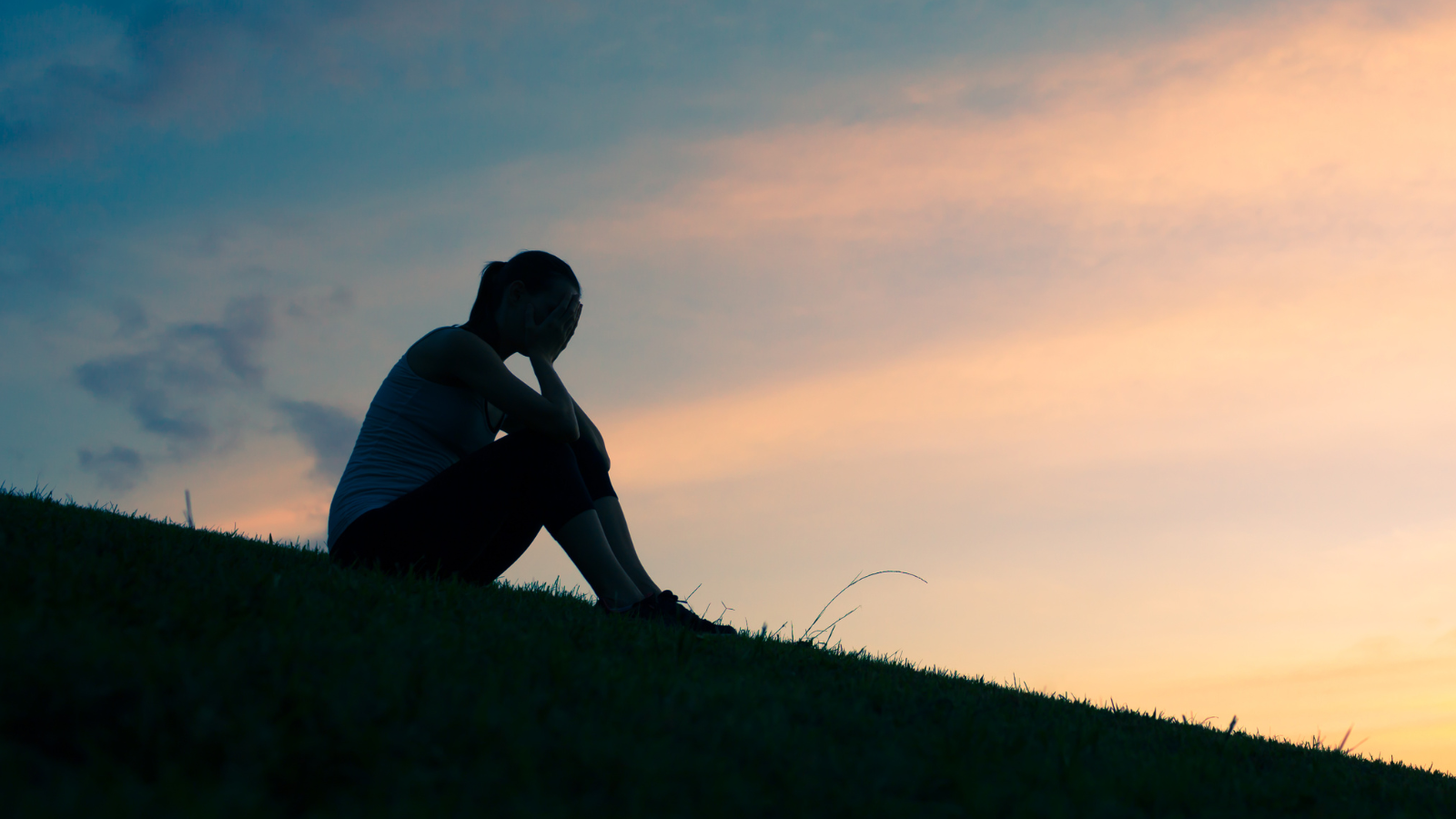
Depression is one of the most prevalent psychiatric disorders globally, and antidepressants are commonly prescribed to patients suffering from depression. However, prior studies suggest that treatment efficacy is usually low. Recent studies aimed to predict antidepressant treatment outcomes but were limited by several factors, including self-reported social information and the types of feature information extracted from patients’ medical records.
In a study in Psychiatric Research and Clinical Practice, Dr. Jyotishman Pathak, chief of the Division of Health Informatics and the Frances and John L. Loeb Professor of Medical Informatics, and colleagues evaluated whether a machine learning approach could accurately predict antidepressant treatment outcomes using electronic health records (EHRs) from patients with depression.
Researchers measured antidepressant treatment outcome over time using the slope of a linear regression model based on all Patient Health Questionnaire-9 (PHQ-9) scores throughout a given treatment period. This model is unlike methods used in prior studies, which typically measure the difference between the final PHQ-9 score and the baseline PHQ-9 score for a certain treatment period. Machine learning models including L2 norm regularized Logistic Regression, Naive Bayes, Random Forest, and Gradient Boosting Decision Tree (GBDT) were also used to predict treatment outcome based on additional data from EHRs.
Results indicate that machine-learned class models can predict antidepressant treatment outcome using patients’ medical history. Results also indicate that diagnostic codes and baseline severity were strong predictors of treatment outcome. The GBDT model achieved the best predictive results. These predictive results can aid clinicians in developing treatment plans, thus accelerating personalized medical management for patients with psychiatric illness.
- Highlights