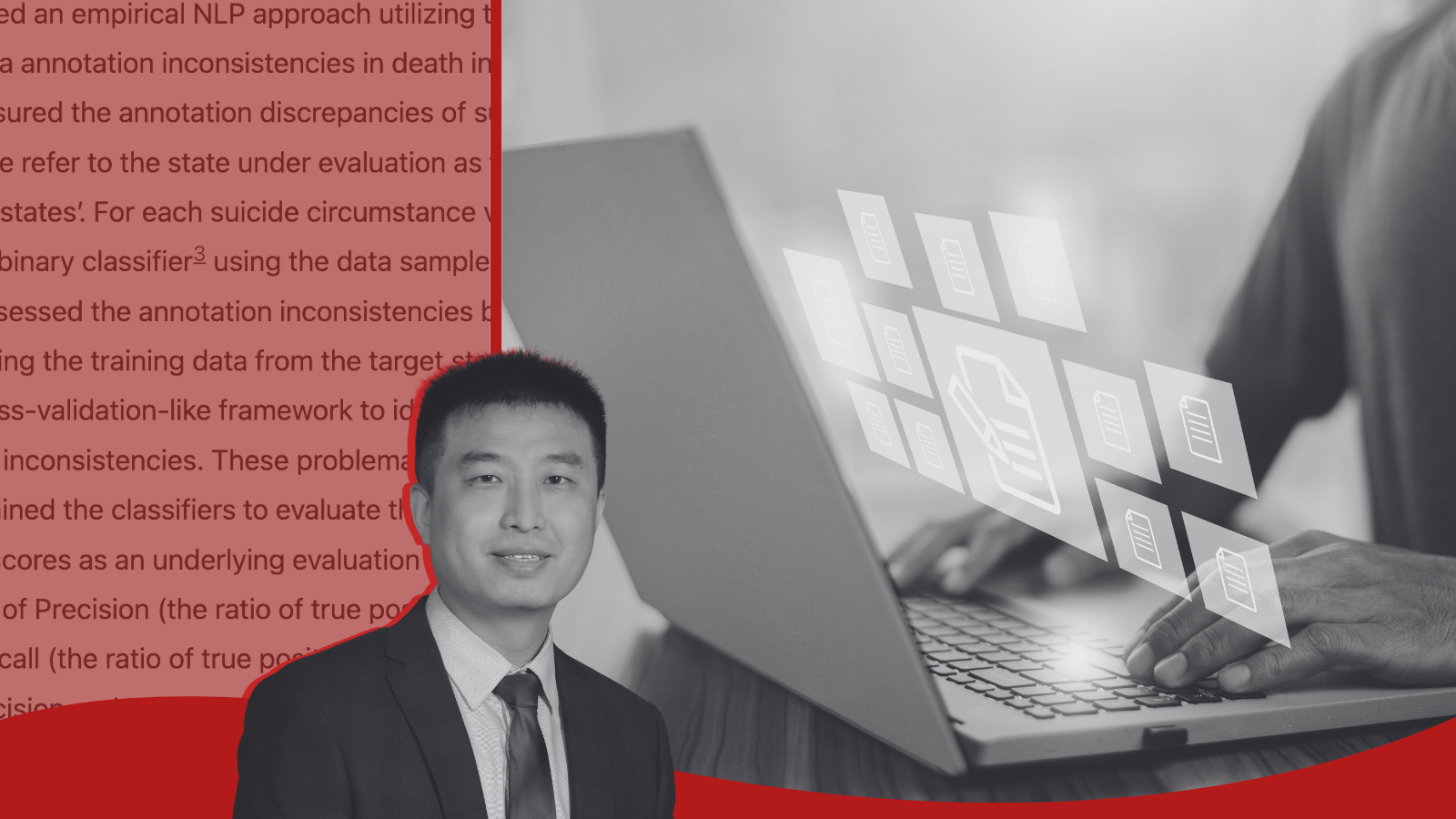
From 2000 to 2021, suicide rates in the U.S. surged by 36 percent. The National Violent Death Reporting System (NVDRS) tracks information related to suicide victims, including demographics and social determinants of health. Currently, NVDRS data is manually annotated by human abstractors. However, the system lacks a peer verification process, leaving room for annotation inconsistencies. These discrepancies pose a risk to the reliability of the data and the conclusions drawn from it.
In a recent study published in Communications Medicine, Dr. Yifan Peng, associate professor of population health sciences, and colleagues aim to address this growing concern by developing a natural language processing (NLP) approach to detect potential data annotation inconsistencies in death investigation notes. He worked alongside Dr. Yunyu Xiao, assistant professor of population health sciences, Dr. Yiliang Zhou, postdoctoral associate, and Dr. Cui Tao from the Mayo Clinic, as well as colleagues from The University of Texas at Austin, including Dr. Ying Ding, Dr. Joydeep Ghosh, and Song Wang. Analyzing 267,804 suicide death incidents recorded between 2003 and 2020, the research team applied a computational method to assess the accuracy of NVDRS records.
Their NLP framework proved effective in identifying annotation inconsistencies and resolving possible errors. The framework could be refined to accommodate more diverse data sources. Researchers also advocate for enhanced annotation guidelines to ensure that datasets are more reliable. By improving the accuracy of NVDRS records, the researchers hope their model will lead to more reliable studies of suicide-related factors and, ultimately, contribute to better suicide prevention in the future.
- Highlights