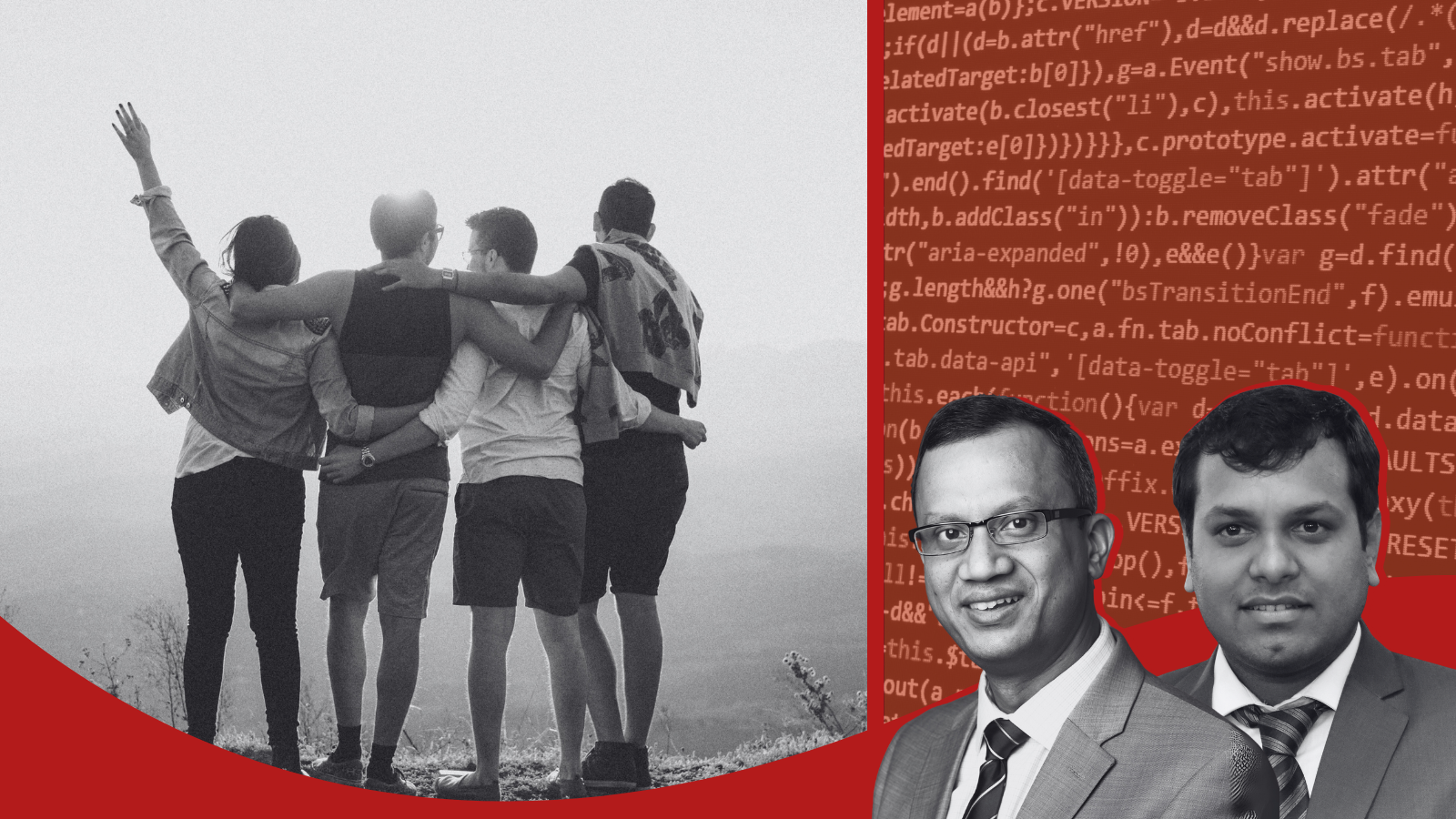
Social determinants of health, including social isolation (SI) and social support (SS), are often associated with psychiatric outcomes. SI is associated with higher health care expenditure, morbidity, and mortality. It is also linked to health risks, including anxiety and depression, among others. SS and related constructs such as social networks and emotional support are associated with improved health outcomes. While both are significant predictive factors, research on identifying SS and SI from electronic health records (EHRs) is lacking.
In a study in the Journal of the American Medical Informatics Association, Dr. Braja Patra, research associate in population health sciences, Dr. Jyotishman Pathak, chief of the Division of Health Informatics and the Frances and John L. Loeb Professor of Medical Informatics, and colleagues developed natural language processing (NLP) systems to identify SS and SI in clinical notes of psychiatric patients and dissect them into distinct categories. These categories include the presence or absence of social networks, instrumental support, emotional support, and loneliness.
Researchers used data from Mount Sinai Health System and Weill Cornell Medicine to develop the NLP systems, which in turn are intended to be portable across these sites.
The study offers both rule-based (RBS) and large-language model (LLM)-based NLP systems to identify categories of SS and SI. The performance of the RBS outperformed the LLMs across all metrics, potentially because the RBS closely follows the rules of a manual annotation rule book. However, the LLM was more inclusive with categorization and allowed for common English-language understanding. Given their individual limitations and advantages, both systems would benefit from additional replication studies.
- Highlights